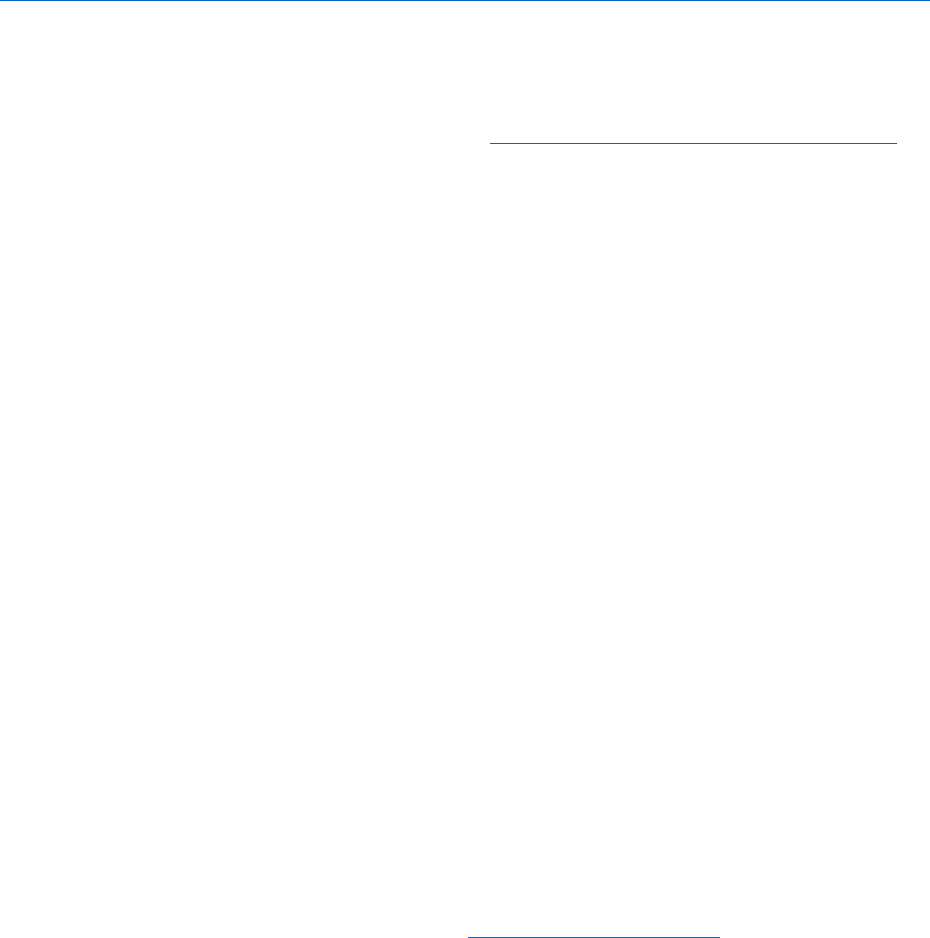
63
Mamun, S., Castillo-Castillo, A., Swedberg, K., Zhang, J., Boyle, K. J., Cardoso, D., Kling, C.
L., Nolte, C., Papenfus, M., Phaneuf, D., & Polasky, S. (2023). Valuing water quality in the
United States using a national dataset on property values. Proceedings of the National Academy
of Sciences - PNAS, 120(15), e2210417120–e2210417120.
https://doi.org/10.1073/pnas.2210417120
Macbeth, E. J. (1989). The relationship of shoreland zoning elements to the aesthetics of
developed lakeshores in Wisconsin (Doctoral dissertation).
Mierzynski, M. (2017). The Many Faces of Water,
http://www.themanyfacesofwater.eu/index.php/2017/05/19/measuring-water-clarity-with-secchi/
last accessed 1 May 2024
Mooney, S., & Eisgruber, L. (2001). The influence of riparian protection measures on residential
property values: The case of the Oregon Plan for Salmon and Watersheds. The Journal of Real
Estate Finance and Economics, 22(2–3), 273–286. https://doi.org/10.1023/A:1007899716050
Moore, M. R., Doubek, J. P., Xu, H., & Cardinale, B. J. (2020). Hedonic price estimates of lake
water quality: Valued attribute, instrumental variables, and ecological-economic
benefits. Ecological Economics, 176, 106692.
Munsch, S. H., Cordell, J. R., & Toft, J. D. (2017). Effects of shoreline armouring and overwater
structures on coastal and estuarine fish: opportunities for habitat improvement. Journal of
Applied Ecology, 54(5), 1373-1384.
Netusil, N. R. (2005). The effect of environmental zoning and amenities on property values:
Portland, Oregon. Land Economics, 81(2), 227-246.
Nicholls, S., & Crompton, J. L. (2018). The contribution of scenic views of, and proximity to,
lakes and reservoirs to property values. Lakes & Reservoirs: Research & Management, 23(1),
63-78.
Otto, D., Monchuk, D., Jintanakul, K., & Kling, C. (2007). The economic value of Iowa’s natural
resources. Iowa state University.
Papenfus, M. M., & Provencher, B. (2005, November). A hedonic analysis of environmental
zoning: Lake classification in Vilas County, Wisconsin. In Unpublished manuscript, University
of Wisconsin-Madison. Retrieved from http://www. aae. wisc. edu/seminars/papers/2005%
20Fall% 20papers/AppEcon/provencher (Vol. 12).
Parkyn, S. M., Davies‐Colley, R. J., Halliday, N. J., Costley, K. J., & Croker, G. F. (2003).
Planted riparian buffer zones in New Zealand: do they live up to expectations?. Restoration
ecology, 11(4), 436-447.
R (n.d.) The R Project for Statistical Computing https://www.r-project.org/ last accessed 31
January 2024